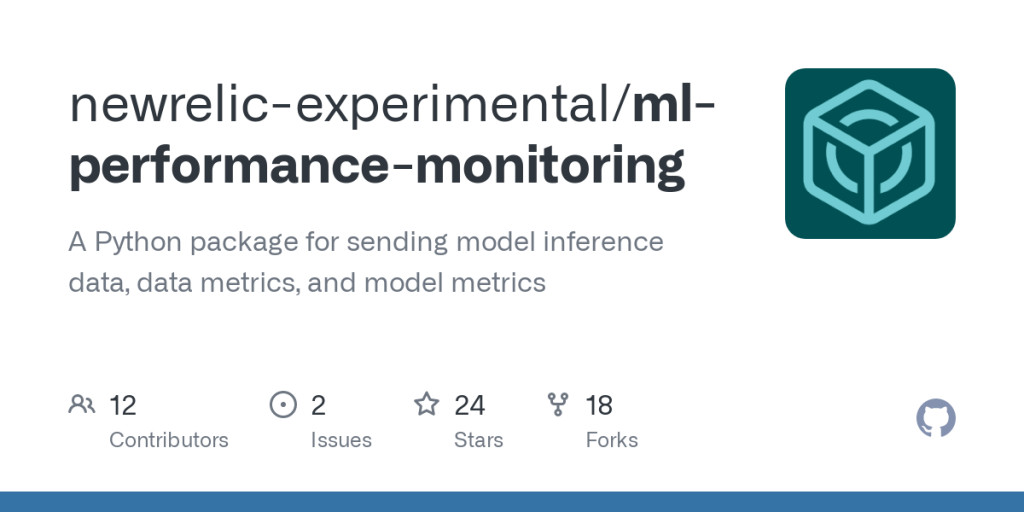
Are you curious about how NewRelic is utilizing MLOps in monitoring and observability? Well, you’ve come to the right place. In this blog post, we’ll dive into the world of MLOps and how NewRelic is using it to improve their monitoring and observability.
What is MLOps?
Before we get into how NewRelic is using MLOps, let’s first define what it is. MLOps is a set of best practices for managing the lifecycle of machine learning models. MLOps helps to streamline the process of training, deploying, and updating machine learning models.
MLOps is a relatively new field that has emerged due to the growing demand for machine learning models in various industries. MLOps is closely related to DevOps, which is a set of practices for managing the lifecycle of software applications.
How is NewRelic using MLOps?
NewRelic is a leading observability platform that helps organizations monitor their software applications and infrastructure. NewRelic has been using machine learning models for quite some time to improve the accuracy of their monitoring and observability tools.
NewRelic has recently started using MLOps to manage the lifecycle of their machine learning models. This has helped them to improve the efficiency of their machine learning operations and reduce the time it takes to deploy new models.
Benefits of Using MLOps in Monitoring and Observability
Using MLOps in monitoring and observability provides several benefits. Let’s take a closer look at some of them.
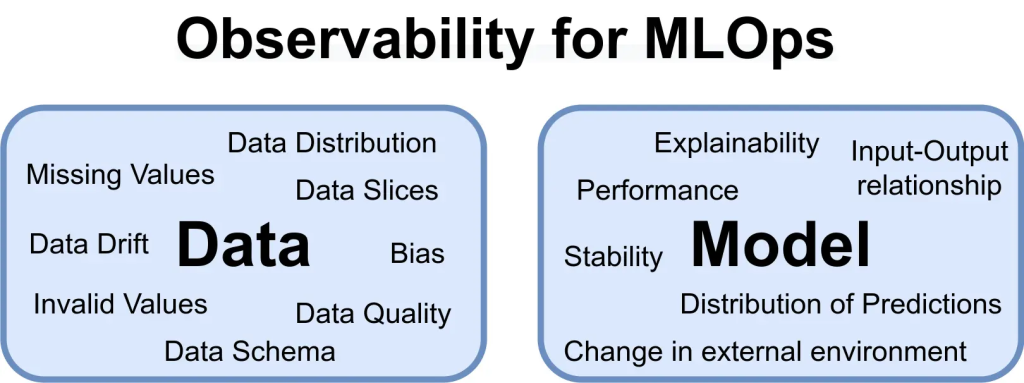
Faster Deployment
MLOps helps to streamline the process of deploying machine learning models. This results in faster deployment times and faster time to market for new features and functionalities.
Improved Scalability
MLOps helps to improve the scalability of machine learning models. This means that organizations can handle more data and more complex models without sacrificing performance.
Better Accuracy
MLOps helps to improve the accuracy of machine learning models. This means that organizations can make better predictions and detect anomalies more accurately.
Reduced Costs
MLOps helps to reduce the costs associated with managing machine learning models. This is because MLOps automates many of the tasks associated with managing machine learning models, reducing the need for manual intervention.
Conclusion
In conclusion, NewRelic is using MLOps to improve their monitoring and observability tools. MLOps provides several benefits, including faster deployment, improved scalability, better accuracy, and reduced costs. As machine learning continues to play an important role in various industries, MLOps will become increasingly important for managing the lifecycle of machine learning models.