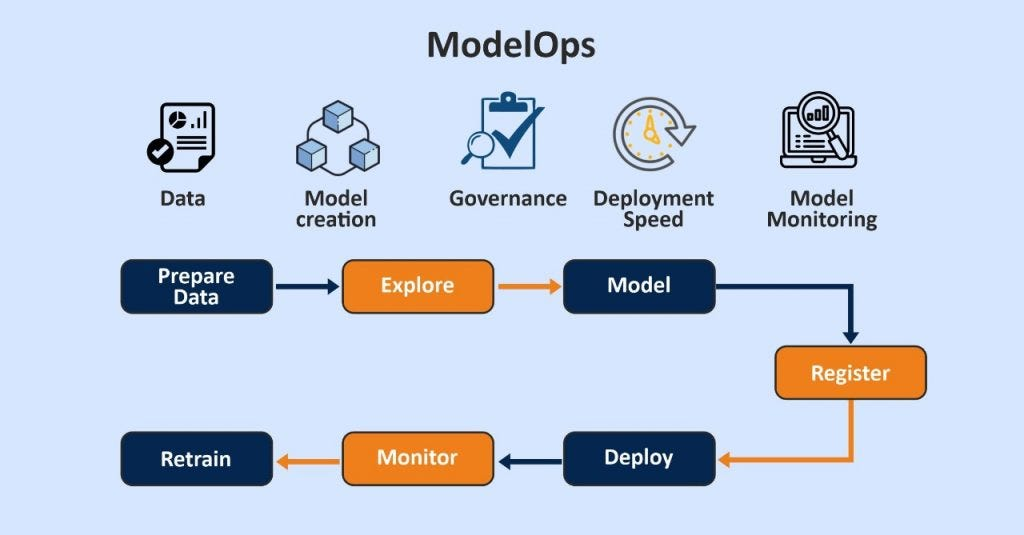
Have you ever wondered about the difference between MLOps and mlops? In this blog post, we’ll explore the nuances of these two terms and help you understand the key differences between them.
The Basics of MLOps and mlops
Before we delve into the differences between MLOps and mlops, let’s first define what each of these terms means.
MLOps
MLOps stands for “machine learning operations.” It’s a set of practices that are designed to help organizations manage and deploy machine learning models at scale. MLOps involves a range of activities, including model training, model deployment, model monitoring, and model optimization.
mlops
On the other hand, mlops (written in lowercase letters) refers to the use of machine learning in operations. This term is often used to describe the use of machine learning to automate various operational processes, such as inventory management, supply chain optimization, and predictive maintenance.
The Key Differences Between MLOps and mlops
Now that we have a basic understanding of what MLOps and mlops are, let’s dive into the key differences between these two terms.
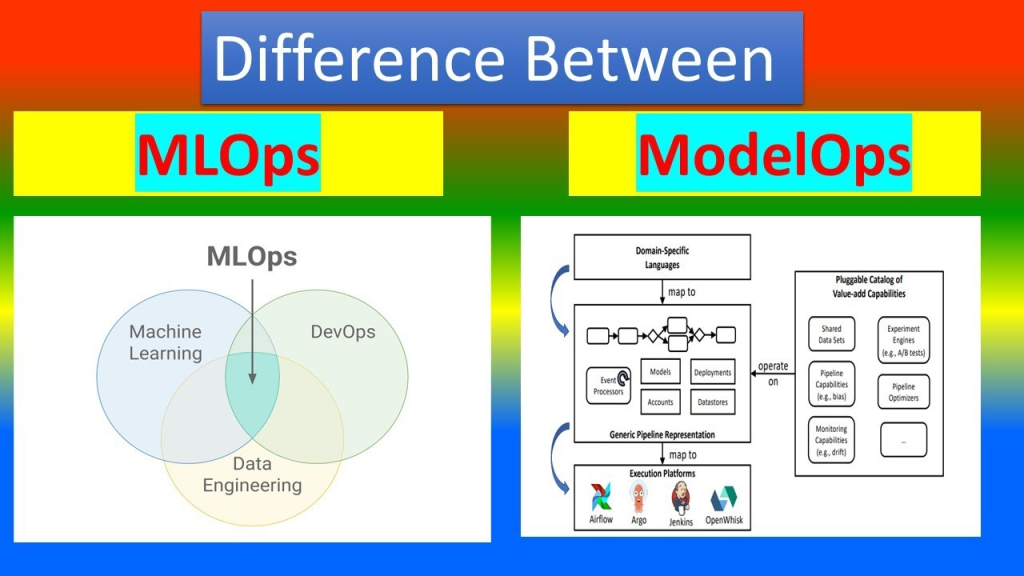
Focus
The first major difference between MLOps and mlops is their focus. MLOps is primarily focused on the management and deployment of machine learning models. In contrast, mlops is focused on using machine learning to optimize operational processes.
Scope
Another key difference between MLOps and mlops is their scope. MLOps is typically focused on a specific machine learning model or set of models. In contrast, mlops is often used to optimize a wide range of operational processes across an organization.
Skills Required
The skills required to implement MLOps vs mlops also differ. MLOps typically requires expertise in machine learning, software engineering, and DevOps. In contrast, mlops requires expertise in machine learning, operations management, and data analytics.
Tools and Technologies
Finally, the tools and technologies used in MLOps vs mlops also differ. MLOps often involves the use of tools such as TensorFlow, PyTorch, and Kubernetes. In contrast, mlops may involve the use of tools such as Apache Kafka, Apache Flink, and Apache Spark.
Conclusion
In summary, while MLOps and mlops may sound similar, they are actually quite different. MLOps is focused on managing and deploying machine learning models, while mlops is focused on using machine learning to optimize operational processes. The skills required, scope, and tools used in each of these areas also differ. By understanding these differences, you can better determine which approach is best suited for your organization’s needs.