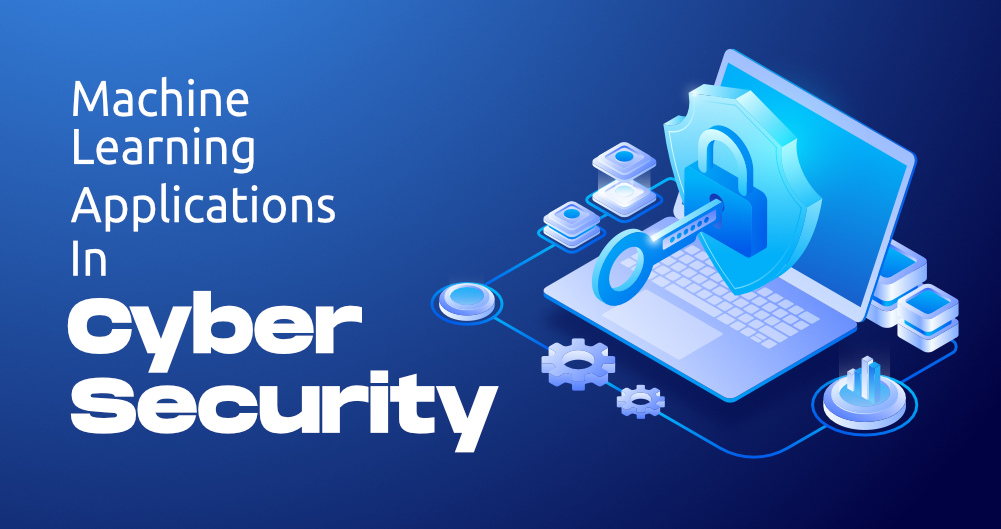
Are you worried about the increasing number of cyber-attacks in today’s digital world? Do you want to protect your organization from potential threats? If yes, then you need to understand the benefits of MLOps in cybersecurity.
Introduction
MLOps refers to the combination of machine learning (ML) and DevOps practices, which streamlines the machine learning lifecycle. It helps in the deployment, management, and monitoring of ML models. MLOps can be a game-changer in cybersecurity as it can help organizations detect and prevent cyber-attacks in real-time.
The Importance of MLOps in Cybersecurity
MLOps can provide several benefits to organizations in the field of cybersecurity. Here are some of the key advantages:
Real-time Threat Detection
MLOps can help organizations detect potential threats in real-time. With the help of ML models, MLOps can analyze large amounts of data and identify patterns that may indicate a cyber-attack. This can help organizations take immediate action to prevent the attack.
Automated Response
MLOps can automate the response to potential threats. With the help of ML models, MLOps can identify the type of attack and provide an appropriate response. This can save a lot of time and effort for security teams.
Continuous Monitoring
MLOps can help organizations monitor their systems continuously. With the help of ML models, MLOps can analyze data in real-time and detect any anomalies that may indicate a cyber-attack. This can help organizations take proactive measures to prevent the attack.
Improved Accuracy
MLOps can improve the accuracy of threat detection. With the help of ML models, MLOps can analyze data more accurately than humans. This can help organizations detect potential threats that may have been missed by human analysts.
Challenges of Implementing MLOps in Cybersecurity
While MLOps can provide several benefits to organizations in the field of cybersecurity, there are also some challenges that need to be addressed. Here are some of the key challenges:
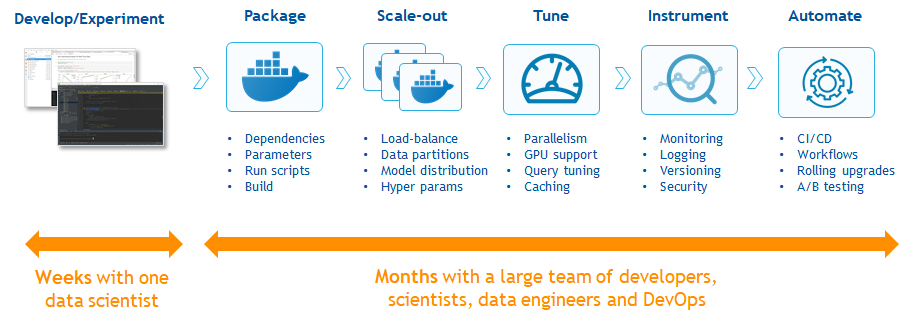
Data Quality
MLOps relies heavily on data quality. If the data is not accurate or complete, the ML models may not be able to provide accurate results. Organizations need to ensure that they have high-quality data to train their ML models.
Lack of Expertise
MLOps requires expertise in both ML and DevOps. Organizations may find it challenging to find professionals who have expertise in both areas. They may need to invest in training their existing staff or hire new staff with the required skills.
Cost
Implementing MLOps can be expensive. Organizations need to invest in the infrastructure, tools, and personnel required to implement MLOps. This can be a significant investment for smaller organizations.
Conclusion
MLOps can be a game-changer in cybersecurity. It can help organizations detect and prevent cyber-attacks in real-time, automate their response to potential threats, continuously monitor their systems, and improve the accuracy of threat detection. However, organizations need to address the challenges of data quality, lack of expertise, and cost to implement MLOps successfully. With the right approach, MLOps can be an effective tool in the fight against cyber-attacks.