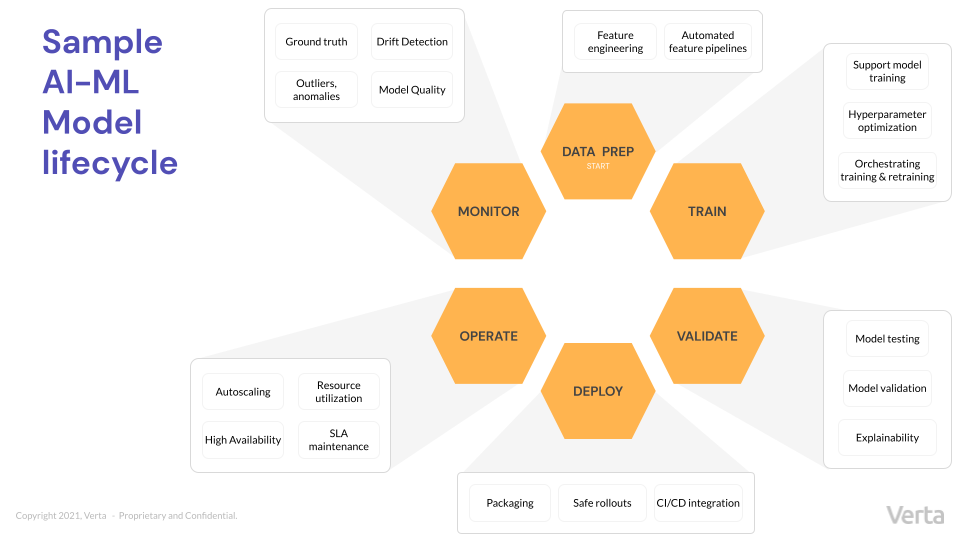
In today’s digital world, data is king. It’s everywhere, and it’s constantly growing. With so much data available, it’s essential to have processes in place to manage, analyze, and utilize it effectively. This is where DataOps and MLOps come in.
DataOps: Managing and Streamlining Data
DataOps is a methodology that focuses on managing and streamlining the entire data lifecycle. This includes collecting, processing, storing, analyzing, and delivering data. The goal of DataOps is to improve the speed, quality, and reliability of data-driven insights.
At its core, DataOps is about collaboration and automation. It involves bringing together cross-functional teams, including data scientists, developers, and business analysts, to work together towards a common goal. By automating manual processes and integrating tools and workflows, DataOps can help organizations become more agile and responsive to changes in the data landscape.
MLOps: Operationalizing Machine Learning
MLOps, on the other hand, focuses specifically on operationalizing machine learning. It’s a set of practices and tools that enable organizations to build, deploy, and manage machine learning models at scale. MLOps is essential for organizations that rely on machine learning to make critical business decisions.
MLOps involves the entire machine learning lifecycle, from data preparation to model training to deployment and monitoring. It’s about creating a repeatable and scalable process that allows organizations to quickly iterate and improve their models. By integrating machine learning into their operations, organizations can gain a competitive advantage and make better, data-driven decisions.
The Intersection of DataOps and MLOps
While DataOps and MLOps are distinct methodologies, they share a common goal: to improve the speed, quality, and reliability of data-driven insights. The intersection of these two methodologies is where organizations can truly unlock the full potential of their data.
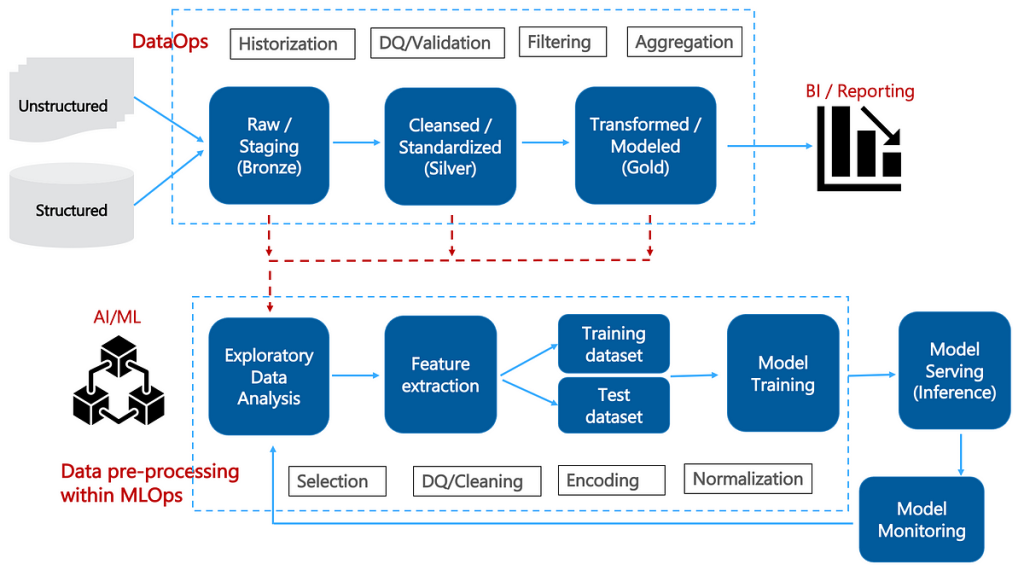
By combining DataOps and MLOps, organizations can create a seamless end-to-end process for managing and utilizing data. This involves bringing together cross-functional teams to collaborate on the entire data lifecycle, from collecting and processing data to building and deploying machine learning models. By automating processes and integrating workflows, organizations can become more agile and responsive to changes in the data landscape.
Conclusion
In conclusion, DataOps and MLOps are essential methodologies for managing and utilizing data effectively. While they have distinct focuses, they share a common goal of improving the speed, quality, and reliability of data-driven insights. By combining these two methodologies, organizations can create a seamless end-to-end process for managing and utilizing data, allowing them to make better, data-driven decisions and gain a competitive advantage in today’s digital world.