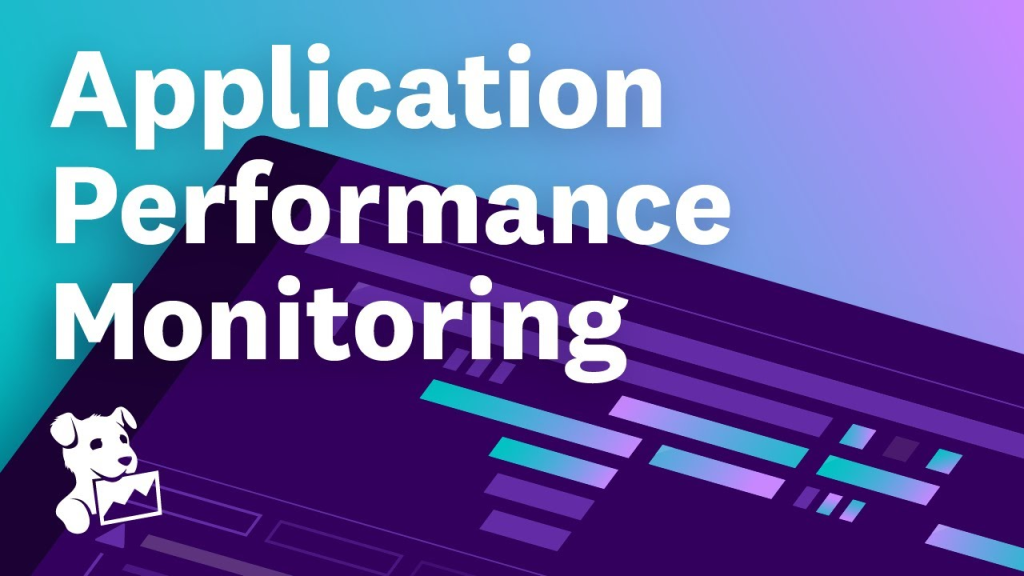
Are you curious about how Datadog is using MLOps in monitoring and observability? Look no further! In this blog post, we’ll explore the fascinating world of MLOps and how Datadog is leveraging it to provide better monitoring and observability solutions for their customers.
What is MLOps?
Before we dive into how Datadog is using MLOps, let’s first define what MLOps is. MLOps, also known as Machine Learning Operations, is a set of practices and tools that enable organizations to develop, deploy, and manage machine learning models at scale. MLOps encompasses the entire machine learning lifecycle, from data preparation and model training to model deployment and monitoring.
How Datadog is Using MLOps for Monitoring and Observability
Now that we have a better understanding of what MLOps is, let’s explore how Datadog is using it for monitoring and observability. Datadog is a cloud-based monitoring and analytics platform that provides visibility into the performance of applications, infrastructure, and logs. With the rise of microservices and distributed systems, monitoring and observability have become more critical than ever before.
Datadog has been using machine learning to power its monitoring and observability platform for several years now. By leveraging MLOps, Datadog can automate the monitoring and observability process, making it faster, more accurate, and more scalable.
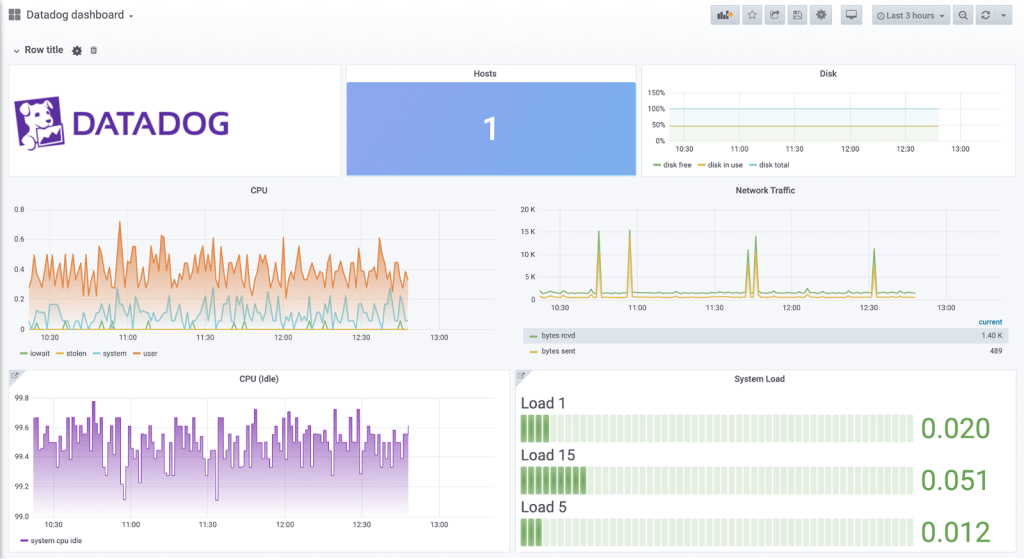
Anomaly Detection
One of the ways that Datadog is using MLOps is for anomaly detection. Anomaly detection is the process of identifying unusual behavior or patterns in data. In the context of monitoring and observability, anomaly detection can help identify issues before they become critical problems.
Datadog’s anomaly detection system uses machine learning to analyze metrics and logs data in real-time. By analyzing large volumes of data, Datadog can identify patterns and anomalies that humans may miss. This allows Datadog to proactively alert customers of potential issues before they become critical problems.
Forecasting
Another way that Datadog is using MLOps is for forecasting. Forecasting is the process of predicting future trends or events based on historical data. In the context of monitoring and observability, forecasting can help predict future performance issues or capacity constraints.
Datadog’s forecasting system uses machine learning to analyze historical data and predict future trends. By forecasting potential performance issues or capacity constraints, Datadog can help customers proactively address these issues before they become critical problems.
Root Cause Analysis
A third way that Datadog is using MLOps is for root cause analysis. Root cause analysis is the process of identifying the underlying cause of an issue or problem. In the context of monitoring and observability, root cause analysis can help identify the source of a performance issue or outage.
Datadog’s root cause analysis system uses machine learning to analyze metrics and logs data to identify the root cause of an issue. By identifying the underlying cause of an issue, Datadog can help customers quickly resolve the issue and prevent it from happening again in the future.
Conclusion
In conclusion, Datadog is using MLOps to provide better monitoring and observability solutions for their customers. By leveraging machine learning for anomaly detection, forecasting, and root cause analysis, Datadog can automate the monitoring and observability process, making it faster, more accurate, and more scalable. As more organizations move towards microservices and distributed systems, the importance of monitoring and observability will only continue to grow. With its innovative use of MLOps, Datadog is well-positioned to meet this growing demand.